It is always a pleasure to participate in the TransELTE conference organized by Eötvös Loránd University, Faculty of Humanities in Budapest.
This year’s edition, titled Professional and Non-professional Language Mediation in the Age of AI was again rich in inspiring talks, this time focusing on the impacts, challenges and risks of using AI in different translation and interpreting scenarios.1
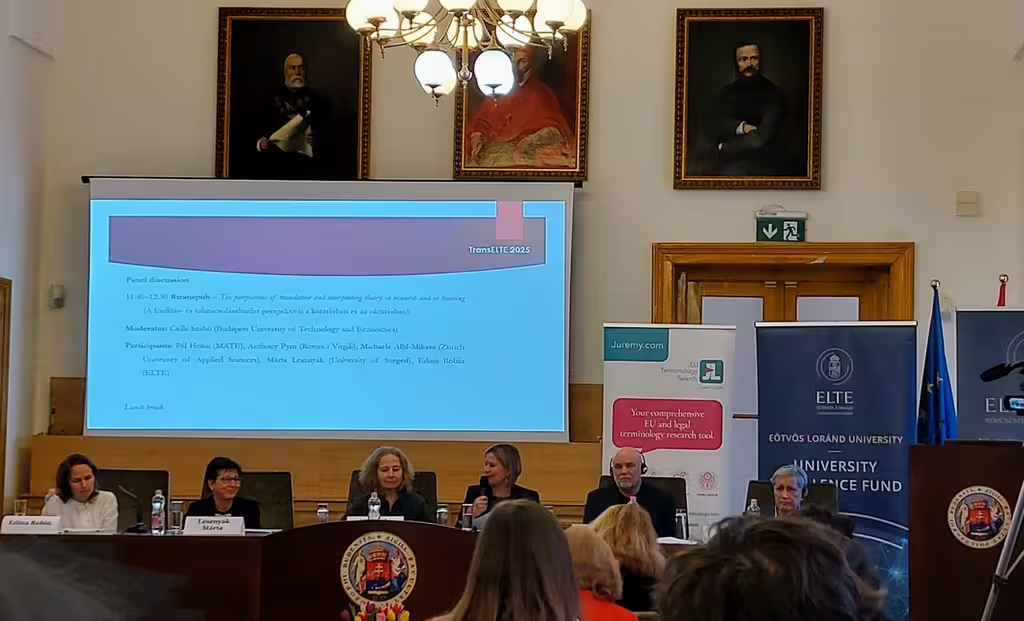
This event is particularly relevant for us, not only because ELTE University is our long-term academic partner, but also because witnessing the increasing focus on AI in different fields of language mediation encourages us to join the discussions by highlighting the importance of quality, terminological accuracy and skilled decision making.
Don’t trust, always verify
Based on the presentations of the conference, it seems generally accepted that we should think critically about AI output and it should be revised by human professionals, especially in high-risk scenarios.
What is much less mentioned is the way how this verification should be done. Everyone agrees that when it comes to high-stake texts, reliability is a key factor. But how can it be ensured?
For us it seems obvious that this skilled verification, which includes terminological decision making and reference research, should be performed with the help of tools which are not based on AI. The reason is this is the only way that the potential errors and unpredictability of AI output can be eliminated.
In the panel entitled “The translators’ skill set in the age of AI”, panelists agreed that AI-tools should be trained on high quality human data. It is absolutely true, but we should also talk about how quality is achieved. How will the AI-output become high quality human data? In our opinion it is just as important to provide tooling for linguists to be able to verify and revise the AI output so we continue to have high quality human translated databases.
In our view, the discussion about translators’ skill set in the age of AI should also include verification and terminology research skills and tools supporting this part of the workflow.
We believe that in domains like legal, institutional or technical fields, linguists should be supported by efficient and specialized verification tooling based on reliable and up-to-date linguistic data. The functionality which we develop at Juremy is designed to support terminology research and also the human verification process “in the age of AI”.
Presentation outlines
It is from this perspective that we highlight the following main thoughts and interesting insights learned from those presentations which we had the chance to attend.
Risk management
Tomás Svobodá discussed the background of genAI, and also the shortcomings which we should be aware of, namely hallucinations and unpredictability. The presentation also highlighted the importance of risk awareness, mainly in connection with confidentiality and data protection. We could learn about the different types of prompting and the impact of prompting to the final output, however, with the disclaimer that its reliability should be questioned.
In his keynote, Anthony Pym focused on the need for risk management skills in the T&I profession. The professor also highlighted the importance of trust: translators need trust in the tools and resources they are using. When applying this to AI systems, the presenter suggests that we should adopt the position of low and vigilant trust.
This is where the role of Juremy can be crucial and where it makes a difference: Juremy only displays trusted and reliable information from the European Union’s Official Journal and the EU’s curated termbase IATE. These are databases which linguists can absolutely trust and thus they can be confident that the terminology they are using can be tracked down and annotated.
Analysis and new developments at DGT
Edina Robin and Szilárd Szlávik presented their research project where they analysed texts provided by EU DGT on diverse topics, including biodiversity, medicines regulators, fiscal policies or plastic pollution. The error types of texts translated by NMT and then post-edited by linguists included accuracy, terminology, linguistic norm and style, according to the DGT’s error typology. The authors emphasized that conducting manual error analysis has been a very time-consuming process where the authors made good use of Juremy to speed up identifying terms and their matching equivalents in EUR-Lex.
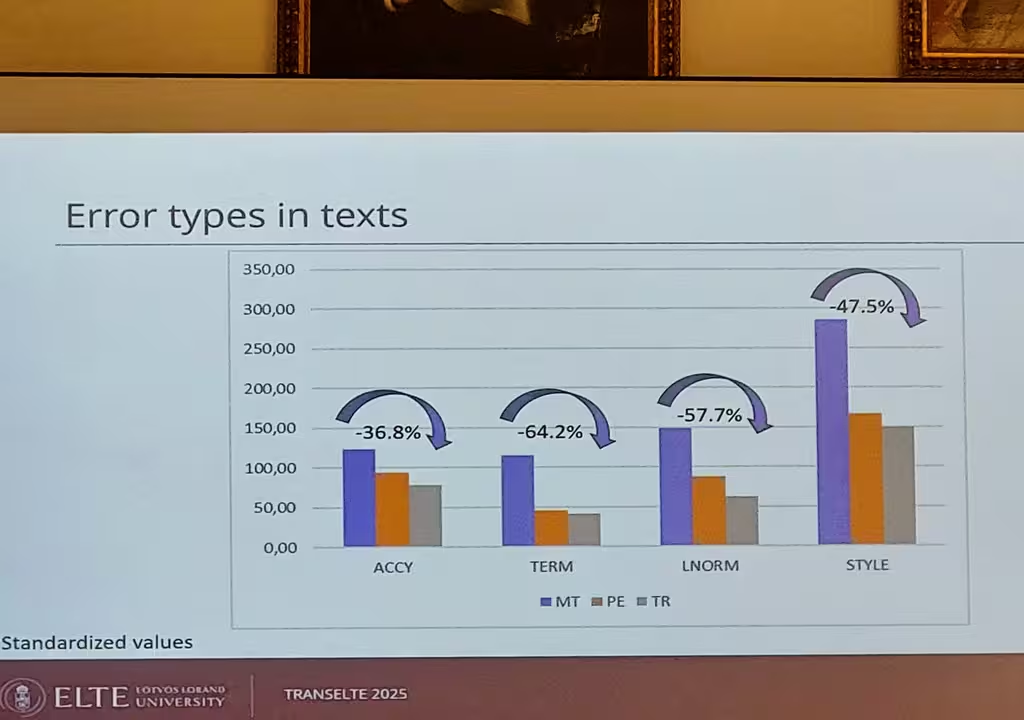
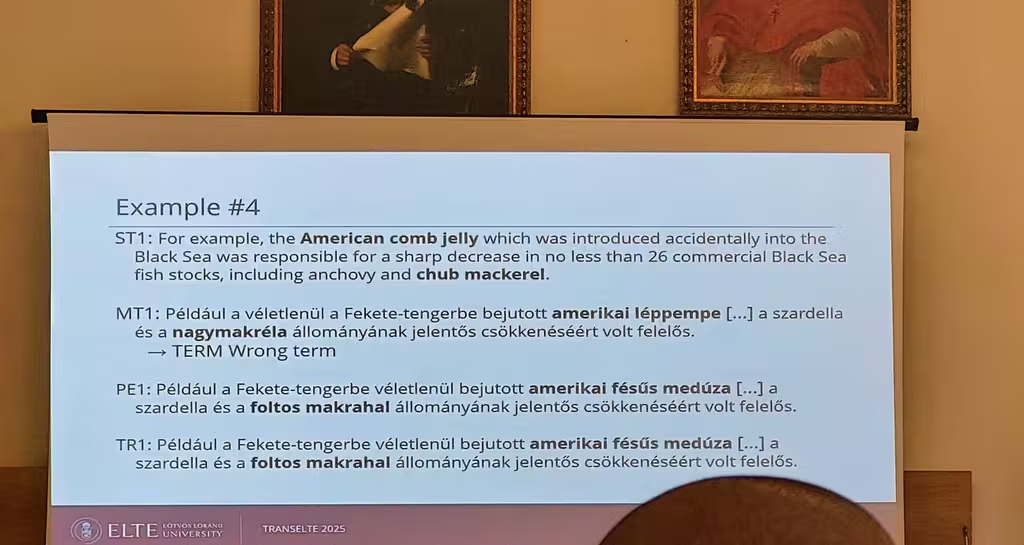
Alenka Unk presented the different linguistic products and services developed by and used at the EU Commission’s DGT. Among all these tools, the presenter called Euramis a goldmine, as MT engines are trained on its data and it only contains high-quality human translations. She highlighted that a new feature has been added to eTranslation: the possibility to upload glossaries in the supported file formats. Alenka also introduced GPT@EC, a secure in-house LLM tool for generating texts using AI. She also shared that terminology is the weakest point of MT and AI tools, as the output often contains inconsistency in terminology.
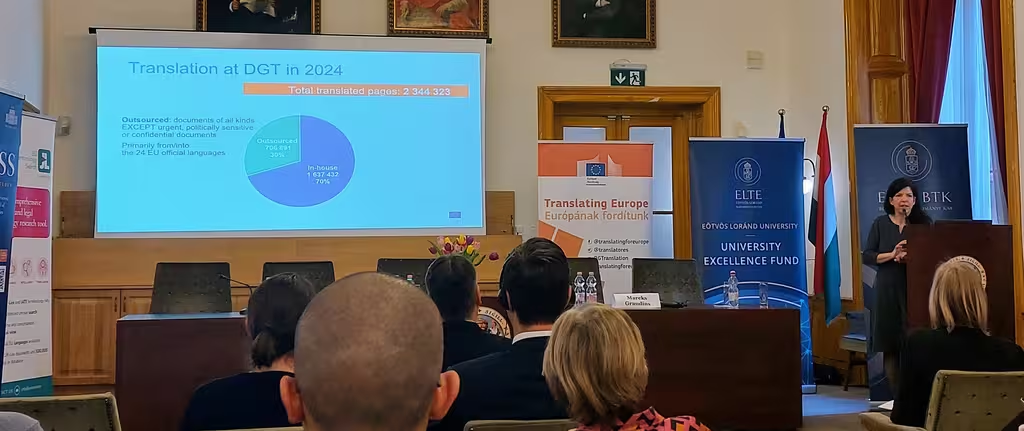
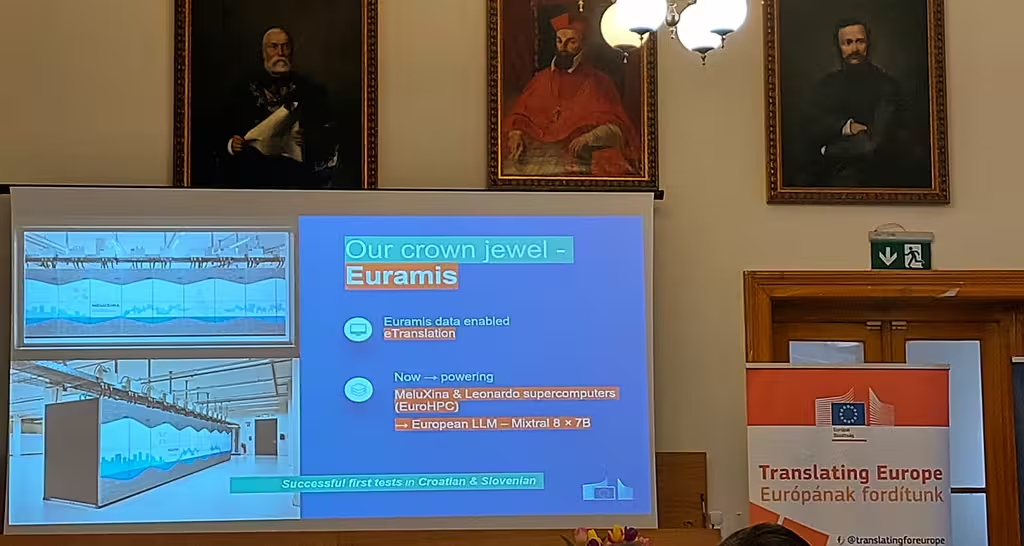
We are very happy that many linguists working for the DGT use Juremy as a reliable companion in improving the terminological consistency and accuracy of AI-based texts, and that Juremy can help them provide high-quality human translations for the EU.
Use of technology tools
Kristóf Móricz and Boglárka Tóth presented their research relating to Technological competence in interpreter training. They also mentioned the study conducted among students about the experiences of using Juremy, which was originally designed for translation use cases, for the interpreters’ workflow. The study found that the students had positive experiences with the tool and were open to using it for preparation to interpreting assignments. The authors highlighted the importance of encouraging young professionals to experiment with new technologies to see if these can benefit their workflow in practice.
Márta Seresi shared with us a case study, where she tested the efficiency of different AI tools in the preparation phase of an EU interpreting assignment related to insolvency law. This subject is rich in EU-specific terminology, which caused problems in most of the cases with regard to the accuracy and terminological consistency of the output.
When pretranslating files related to the assignment, in the case of one of the AI-tools, incorrect or missing terminology was a regular issue. Instead of using specific terminology, the tool explained in very simple terms what was included in the text. All in all the text was very transparent and well-structured, however if you looked at it closely it was incoherent and contained repetitions and other errors.
Another AI-tool which was tested created a translation from the lengthy document very quickly, but similarly to the first tool, it avoided using terminology or misused it. In summary, the presenter found that its capabilities were not suitable for high-level assignments.
Thirdly, Márta tested an AI-tool developed by the European Commission. Although it took a longer time for this tool to provide a translation into Hungarian, and the text was not as structured as in the first two cases, the main notions were well explained, and the Hungarian terminology was mostly accurate. It should be connected to the fact that this tool was developed by the EU and trained on domain-specific EU materials.
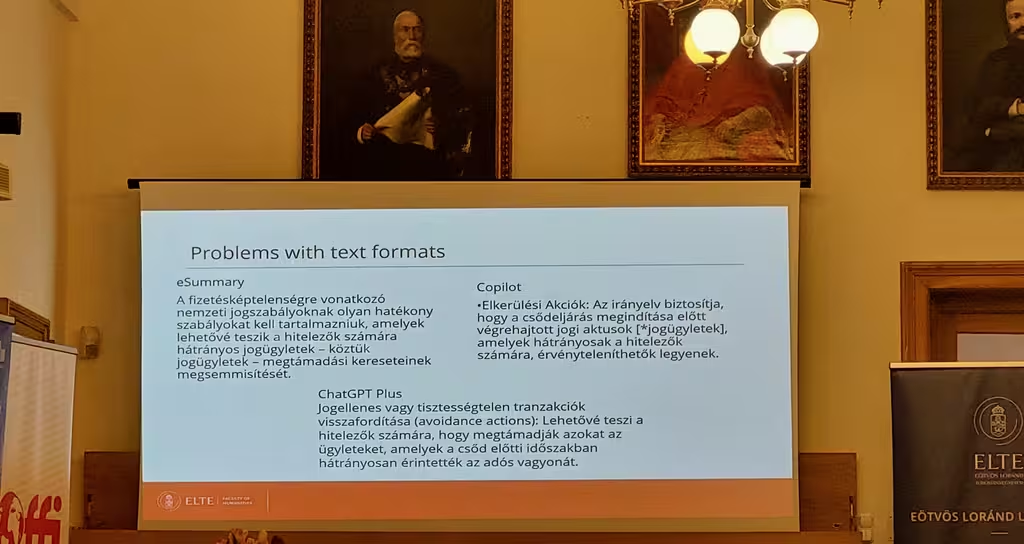
The presenter highlighted the conclusion that if you have an assignment on specialized topics, you need specialized tools.
Márta Seresi also tested these tools for creating glossaries for interpreting assignments. She had the experience that after a while, AI-tools “got bored”, and extracted terms only from the first part of the document. This is in line with Tomás Svobodá’s findings when testing genAI for term extraction functionalities: the tool tends to give up after a while and provides more reliable results only on the top of the list, also it very often invents non-existing terms. In summary, the glossary extraction might work better for general texts, however for long and specialized texts the results are too few and too random.
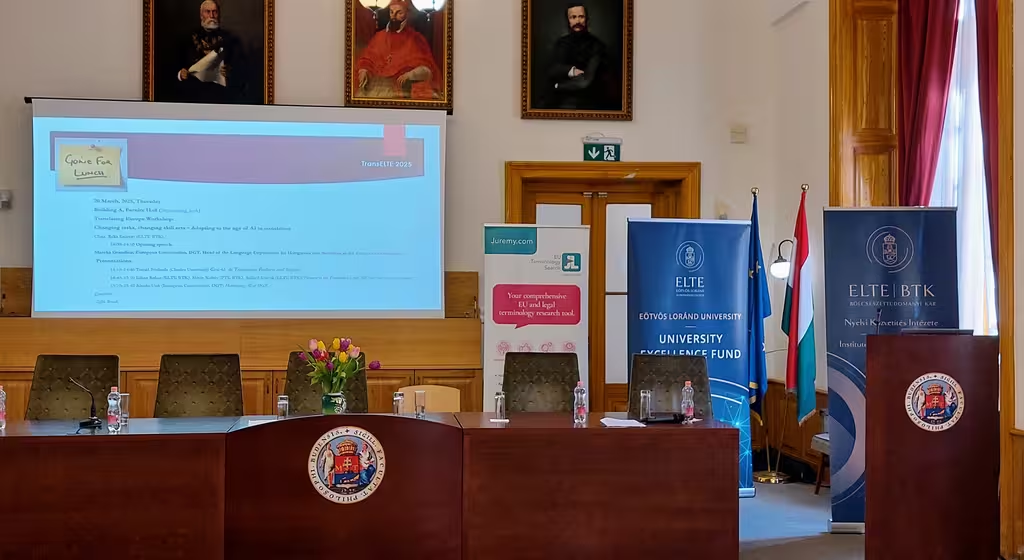
Recap
To summarize the main findings of the presentations learned at the conference:
- The future will be for those who are aware of the advancements and also the limitations of technology.
- When it comes to high-stake texts, reliability is a key factor.
- Handling of terminology is the weakness of most NMT engines.
- AI output sometimes seems too authentic, thus we need critical thinking.
- Translators need trust. Vis-a-vis AI tools we need to take the intermediary position of low or vigilant trust.
- Training information must come from high quality human data.
- General AI tools are very “street smart”, but when it comes to more technical terms they usually fail.
These findings reinforce our belief that domain-specific human expertise, which is the basis of expert research skills, will continue to be indispensable for high-quality and reliable language mediation. This is what we are dedicated to supporting in the future as well.
We would like to thank the ELTE University for organizing this event and providing opportunities to engage in meaningful discussions about the current trends in translation and interpreting.
-
A copy of the programme is available to download in docx format here . ↩︎